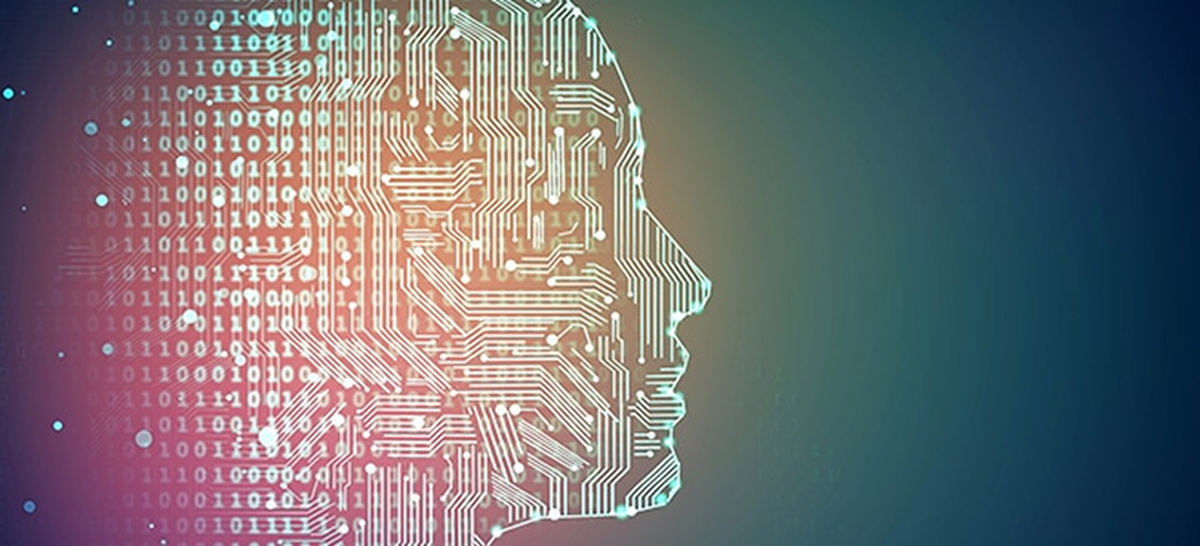
The Case for Banks, Insurance & Transportation Sectors
This article is the second in a series where I explore the implementation of Artificial Intelligence and Machine Learning technologies in Nepal. Some of these can include businesses that are working in this space, based out of Nepal.
Previously I talked about AI/ML tech implementation in three traditional industries in Nepal - the agriculture sector, the education sector, and the health sector.
This time, I want to explore the banking, insurance and transportation sectors since they impact a lot of people in their daily lives.
The Banking Sector
In September 2019, five Chinese nationals were arrested for bank fraud through which they managed to steal Rs. 1.26 crores plus around $10,000 in two days. Police deduced that they had hacked the Nepal Electronic Payment Systems (NEPS) and used both real as well as cloned cards at least 700 times to withdraw millions quickly from the ATMs of various banks. Use of AI/ML would have helped detect and tackle such instances much quicker. The Machine Learning model for this can be trained to identify a range of normal transactional behaviour and historical data for both the specific ATM and the card/account holder. AI systems will have access to this information and any significant deviation in the monetary amounts or number of transactions will be notified to a bank employee who can manually review them. For instance, when hackers want to steal money from ATMs just as in the example above, they will want to maximise the amount they can take out using each card. If they are to withdraw the maximum daily allowed amount, the thieves will have to withdraw money multiple times using the same card. Very few people withdraw the maximum amount during one use and thus if money is withdrawn multiple times using that card, that will be a red flag. The thieves will probably use the same ATM to withdraw money using a few cards and if this pattern repeats on each subsequent withdrawal, that is a bigger red flag for that ATM as such behaviour is highly unlikely to occur. There are additional statistical AI prediction models that can be added to this to predict potential fraud more accurately. In addition to this, the inclusion of facial recognition technology in the surveillance cameras that already exist in most ATM booths can provide an additional layer of security. A potential application is for a face to be matched with a card that is being used. For future such uses, the face and the card can be used, without storing any personally identifying information. When the same person gets detected using multiple ATMs in the same day using multiple cards, this can be identified with this technology.The Insurance Sector
After the 2015 earthquake, many had to face an inordinate amount of delays in their insurance claims for damaged property. A national weekly had reported that many were disappointed in having to wait for a long time, only to then go on to be under-compensated. Insurance valuation and claims processing inherently consumes a lot of time, effort and manpower due to the necessity of physical examinations. Agents have to manually go to each location, examine the damage, make estimates, and then calculate the compensation. Businesses in developed countries are moving towards an analysis and mapping system that is driven by AI/ML. For instance, Cape Analytics is a US company that helps banks and insurance firms to better analyse property risk and condition. They provide them with data using satellite imagery or drone footage to help evaluate risk and identify actual or potential issues. In emergency situations, damage assessment estimation need not be perfect but accurate enough for the company to use. Through multiple drone footage and satellite imagery, an AI/ML system can estimate damage and risk exposure quickly, allowing for swift response times. Even if someone tries to falsify damage assessments, comparison with existing data will expose it. Overall, claims processing will be faster and cheaper, and insurance companies will become more efficient.The Transportation Sector
Autonomous driving technology has been progressing in leaps and bounds, spearheaded by Tesla’s research and investments. It requires lots of data and consistency in traffic rules to be implemented properly in cities and urban locations. This includes lane marking with cameras, traffic lights, signs, etc. As such, I would not expect it to be implemented in the cities of Nepal, or most Asian and African countries, for quite a long time. Highways, on the other hand, can make use of this since the rules are simple, there are no intersections, and the traffic is not as heavy. In the US, a startup company called TuSimple is focusing on autonomous driving tech for heavy-duty trucks to make long haul deliveries. They start out and end with a human driver but an AI takes over all throughout the highway, for hundreds if not thousands of miles. Their self-driving trucks are capable of operating 24 hours a day, 365 days a year in nearly all weather conditions. The trucking industry seems to be the prime candidate to benefit from self-driving AI tech because of its high utilisation and clear value proposition. Here, the majority of truck accidents are due to inexperienced drivers, overestimation of driving skills, hasty decisions, lack of knowledge of the impact of the weight of the load, etc. A self-driving truck with proper AI implementation will provide a much safer and more consistent output albeit at the cost of a slower pace. The human driver will take over in dense areas and outside highways whereas the AI will cruise through the rest. Speed calculations in overtaking as well as identification and avoidance of potential risks will allow for millions to be saved in life and property damages. In the next part of the series, I want to have a look at customer support (spanning multiple industries), robotics and vehicle traffic monitoring.
Published Date: October 14, 2020, 12:00 am
Post Comment
E-Magazine
RELATED Tech Mind