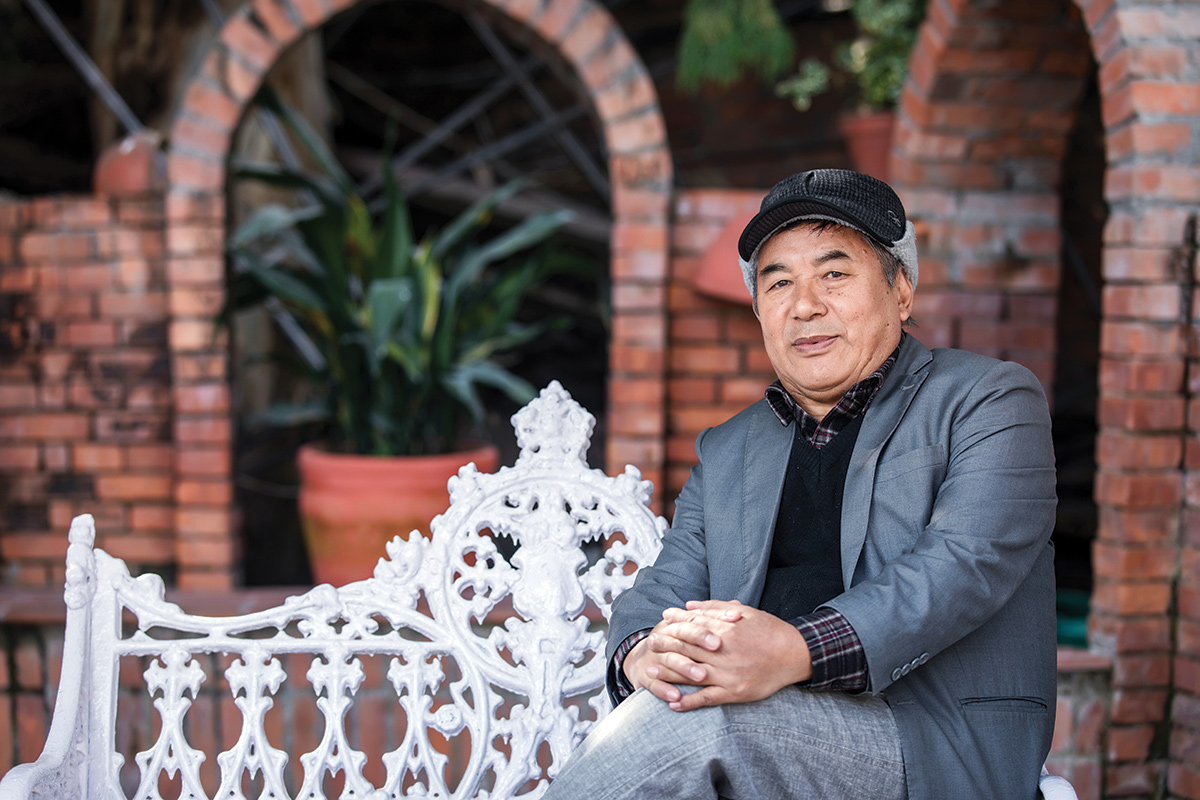
Sameer Maskey is the Founder and CEO of Fusemachines Inc., which is an Artificial Intelligence (AI) solutions and services provider. Fusemachines was founded in 2013 with it’s headquarter in New York. It’s office in Nepal is located in Kathmandu.
On June 26, this year, AI Breakthrough, an independent organisation that recognises top companies, technologies and products in the global AI market, awarded Fusemachines with “Best AI Startup” of 2018 title.
Its founder, Maskey has more than 15 years of experience in natural language processing, machine-learning and data science. He holds a Ph.D., M. Phil. and M.S. in Computer Science from Columbia University. Maskey teaches Data Science and Technology Entrepreneurship at his alma mater. Besides, he has published more than 20 peer reviewed articles and served as Session Chair, Program Committee Member, and Review Committee Member in many international conferences.
We sat down with Sameer Maskey at his Kamaladi office to know about machine-learning, his company, the advancements made in the field of AI, and if we should be wary of the talks around AI causing people to lose their jobs. Excerpts:
Principally, machine intelligence is to augment the intelligence of people, to remove tedious repetitive tasks so that humans can dedicate more time to creative and imaginative endeavors so that they can accomplish more, but the commonly held notion is “Artificial Intelligence possesses an imminent threat to society, capable of possibly leading humanity to its end.” Why is doomsday projected in discourses and especially in movies as a result of AI?
The Terminator Doomsday is not happening any time soon. Machines being intelligent enough that they will rise up against humans is to give way to the notion that machines can survive against humans and that they have consciousness. Reality is, we still do not know what consciousness is. Consciousness, by the way, is one of the defining features of humans. With AI, however, we are not at a point wherein we can say that the machine is conscious. And I think, for a doomsday scenario, we need machines that are conscious and fighting for its life against humans. So I think we are very very far out from there.
I know some big names including Elon Musk have said doomsday is going to come and we will be wiped out of the planet and what not. Having said that, we have to be careful about how AI is used in certain cases for instance, military purposes.
Even though doomsday is far out, the current state-of-the-art technology could be actually misused and threaten humanity in some sense. Somebody could use a drone and say, ‘all the people of this colour, kill them’. And drones as machines, they will not hesitate. That can be done even with the current state-of-the-art technology. It can be done and that’s scary.
So even though conscious machines rising against humans is not going to happen anytime soon, but machines being modified, even with the current state-of-the-art, to do wrong is quite possible and that could actually threaten humanity.
It is argued that with AI being part of our lives, it will cost many people their jobs. Is there any substance to this fear? Are machines eventually replacing humans? Should we fear the rise of machines?
So doomsday scenario regarding AI was one aspect and then there’s the other part about people losing jobs as a result of AI. That is going to happen. That is already happening and it’s going to happen more. It’s because there are a lot of tasks that are repetitive in nature which do not require a lot of thought process. I am talking about tedious repetitive jobs. Those kinds of jobs could be taught to machines such that machines can learn how to do it and actually do it.
We can take the example of a call center. If a company has a new scheme, then people would call up to know about it asking the same kind of questions. And one of the reasons call centers have the highest attrition rates on jobs and one of the lowest job satisfaction is because it is a mind numbing job, you have to answer the same thing over and over again. But we can build machines that can understand humans to some degree, especially if it is related to one particular topic and be able to answer the question, which means machines don’t get tired, they don’t need food, machine can talk to 2,000 people at the same time, and when that happens, people are going to lose jobs at call centers.
This is just reality!
But what about handling new question, new problems—something the machine is not familiar with?
Basically for a machine, if an information has not been fed into it, it does not really know about it. The ease with which a human is able to take disparate sources of information together to come to some sort of decision making process and synthesis and be able to answer a synthesised answer, is little hard for machines. That is there. So when a question about something completely new is asked; something a machine absolutely does not know anything about, then it won’t be able to answer that at all. However, even humans may not be able to answer if it is something new that they do not know about.
The one way to address that in the machine-learning systems is basically to have some sort of self-learning mechanism where if it does not know the answer, it could ask a human and the human would answer it and if the human answers even once, it can learn from it and answer it the next time if it is asked.
Jeff Dean, a Senior Fellow in Google’s Research Group, in his TedTalk session said, “AI is going to be more impactful than the invention of personal computer, and mobile phones.” How and in what ways?
There is a couple of things that have happened, sort of together, and have some sort of alignment where it is now possible for machines to do a bunch of things even better than humans. For e.g. image classification system, in which if an image is shown to a human, and is asked, ‘what is it?’, current accuracy for humans is, if you are given 100 images, you correctly categorise 95 of them, that means 5% error and 95% accuracy. But we are at a point where a machine’s deep-learning base system—image classification system is lower than human error rate which is almost 97% accuracy and only 3% error rate. We call that a super human performance i.e. it is better than a human on a given task.
Now that happened for a couple of reasons: We are at a point where we have so much data for any given task, especially in the last 10 years. We are collecting more and more data and people have been storing more and more data because the cost for storage of data is becoming cheaper.
At the same time, the research on the computational power, the CPU is also doubling. Moore’s Law which was formulated around 1970, stating that the speed of CPU will double every two years, still holds true today. So that means it can now store all these data for any task and there’s the computational power to process all of it. With the advent of deep learning, with its multiple layer based algorithm, it can take all the data and extract features and patterns against all the data search. This has allowed machines to sort of figure out basic pattern interaction and dependencies such that it is able to predict something very accurately.
So we are at this point where three things happened: algorithm, data and computational power. It is because of that, machines started to do very well at pretty much all the tasks that a human does — image classification, video classification, object detection, question-answer system, summarisation, machine translation, speech-to-speech translation, broadcast news transcription, sign language translation.
Everything we as humans do, pretty much all the tasks, if it can be automated, then it is automated. Coming to Jeff Dean, I suppose what he is saying is that, basically most tasks can be automated. What cannot be automated is love, passion, relations but as we are humans that is one part of it but in day-to-day job, it’s the function of what we do as a task and if that task can be automated, and if most of the tasks out there in the world that we do on a daily basis in an office can be automated, that means it is going to disrupt pretty much every field.
Let’s come to your company Fusemachines. What new and different does it bring to the existing system? What areas of AI is it in?
We have a couple of products. We have a product on AI for Customer Service. New York City Government was our first client where we saw it being deployed to improve customer service because a lot of people did not have to wait on the phone for like 20 minutes to get an answer. Customer service automation is one of the big piece products.
The second product line is AI for Sales wherein we have machines ‘listen’ to the web and track people and companies. That way, machines figure out if somebody is trying to buy something. They track people and companies and figure out the buying intent. It is basically a sales automation tool so that sales people have to do less work. The machine will do most of the work for the sales person in finding leads and responding to them and so forth.
The third product line is the AI Learning Platform which allows a lot of people to learn AI and that is one of the big themes in our company right now. I am an educator and I have seen how education makes a lot of difference in people’s lives and I have seen the capability of my students at Columbia where I teach. But I also know that there are many capable students in Nepal who might not have got an opportunity to learn. So we have created this AI platform where they can learn AI. We also run fellowship programs called Fusemachines AI Fellowship.
Besides those products, we have a service line of business that we call AI as a Service. What we do with it is, we train young engineers in Nepal, New York, Dominican Republic, Rwanda, and the new countries that have been added: Jamaica, Romania and Peru. So all these underprivileged kids, and even in NY (we visit lower tier schools where there are good students but who could not afford the school because of monetary issues), we train through our fellowship program.
We focus on underprivileged engineers, train them, groom them and get them ready to build industry products. And when they get ready, we hire them and – sort of half of them — we have them work in our set of products and other half, we provide them as a service to other companies that are looking to hire AI talent. And that is AI as a Service.
Why Rwanda, Romania, Peru? Why did you pick these places?
Especially, in the beginning, our criteria were mainly “Are there enough talent in this country?”, “Can the talent be groomed?” and “Is it a developing country where they might not have got an opportunity even though they have talent?” But lately, even though in the strictest sense, it is not a criterion, but we also make sure that the young talents also speak English, to some degree, because all the learning, everything is in English.
So, basically, we pick a developing country where there is talent.
So how much is Fusemachines investing in the entire Fellowship Program and grooming process? What sort of return is it looking at?
We started the Fellowship in January 2017. When we do each fellowship, we select 25 students for each cohort. Per student we spend about $1300 just on fees. And then there are additional costs. All in all, for each cohort, in each city, we spend about $40,000-$50,000. And we do it across many cities at this point. We are investing quite a bit. We used to do it once a year but now we are doing it every six months across all cities. So the cost does add up.
We started doing this not from the perspective of solely making money. We started in Nepal and the idea was to enable a lot of Nepali kids learn AI, get them excited about it and start building AI things for Nepal itself.
When we first opened up the Fellowship, we had hundreds of applicants, I think about 500 applicants or so and we selected 25. We were very strict about the selection process, exclusively merit based. But we discovered that almost 10 of the selected ones were from different companies. We were in a fix: if we do select just 25, just based on the merit as we had said, we would be essentially spending significant chunk of cash to train somebody else’s employees. But we stuck to our words and we trained everyone who are at different companies at this point.
But what seemed to help was it actually helps on the bigger purpose of AI being more interesting for more companies, more people and more kids getting interested. Now, at this point, we select 25 - no matter how many people are from other companies, we still train them. But usually half of them are graduating students - those are the ones we hire and, eventually, they become our engineers.
So now, AI Fellowship has become a sort of sourcing place for us, and finding the best talent. And because it has become quite popular, lots of kids are starting to change their LinkedIn account and say FuseMachines AI Fellow. It is because the Fellowship has become so popular, we are able to narrow down the pool, which means we are able to get, literally the best and the brightest of all the graduating kids.
Is Fusemachines an outsourcing hub? Do you cater to local companies here in Nepal?
We have not catered to any local companies. We have not done absolutely any business with any local company. We stay like that partly because the scale of things is different. The contract sizes here in Nepal are very small; and from what I hear, it’s hard to get paid on time; and that even after getting contracts, one has to deal with too many stuffs. We don’t want to deal with that. But too many inbound clients pursue us as they want to work with us but we have been saying no.
However for the first time, we are about to take a Nepali client. So we are starting to take Nepali clients.
Why should the world -the non-Silicon Valley population - be interested in AI and how will AI affect our future?
How AI can impact a country like Nepal. In a bunch of ways: 1. Agriculture. 2. Delivery of medicines 3. Besides, agriculture and medicine, there are other used cases. We have been building a Character Recognition System that takes a form (written in Nepali) and fills it up for you. Right now if you go to banks to open an account, you have to write everything. And then a person would take that form and start typing everything. Now, that is inefficiency. So what we have been building is a system that we think has too many uses. 4. We are also working on Nepali Speech Recognition System and now it can recognise digits and words which means it will be useful for blind people.
These are the things I am proposing for Nepal.
Are most of the projects targeted for a fixed geography?
Not that different actually. So there are products that we have built for US and the European market. And then there are research projects that we are doing for developing countries that could translate into products. The research projects are not fully products yet but they are starting to become products. Those research projects made for developing countries are not made for a particular country but it’s applicable across all the countries in a very similar way if they have similar problems.
The medicine carrying drones and agriculture, there are other companies that provide the same service, how does one differ from the other?
There could definitely be more than one country trying to do the same, that’s just business. And the way to compete with one another is to see who builds the better product with better set of features.
One thing that is not easy in AI is building well designed working system. It is easy to make a sort-of working system, but improving the accuracy from 50% to 60% to 95%, is difficult. And that basically becomes one of the key differentiating factors between a cutting edge AI company and another AI company that is based on open search tools and just using the libraries and does not know the in-depth of the math. At the very basic of it, AI is all math combined with programming. And because it is math, a lot of engineers are not actually as good as they should be in machine-learning. They know programming, they might be amazing programmers, but to be a really good machine-learning engineer, you also need to know math very well. You need to know linear algebra, calculus, probability and statistics. If you have your foundation right, you are bound to build a better product than the other companies.
You said it in your article, published in Forbes, “As artificial intelligence permeates through every field in every industry, there is a war for AI talent. In fact, Silicon Valley giants are fighting and paying an exorbitant amount of money to lure the best AI engineers to work for them?”… Could you elaborate.
People have come to the realisation that there’s this kind of technology that is going to disrupt the way the world is going to work. Just imagine, when self-driving cars will become fully real. By the way, self-driving car is very close to real at this point.
Now if AI is becoming disrupting to the point that it is going to completely change the auto industry, then there are a lot of other industries that are going to look different. For e.g. manufacturing.
All countries are starting to realise that if they want to be competitive in the next modern world, then they have to bet on AI. And I think that is what China has done and China is probably outdoing the US at this point.
How do you see the race for AI between the USA and China and everybody else wanting to get in?
One thing to consider is, there’s way too much hype. This has happened before as well. There’s this concept called “AI Winters” where AI becomes so popular and everybody thinks AI will change the world and then nothing really happens. And then because people start to realise it is just a lot of hype and there’s not a lot of reality around it, slowly the funding starts to dry out and resultantly, the AI industry starts to dry out. And that’s AI Winter where there is not much research done. So if you see, there have been a couple of AI Winters already.
In that regards, some people predict that the next AI Winter is impending. But I think, the case is slightly different this time around. I think a lot of it is real. Having said that, there’s still way too much hype where a lot of people who actually don’t know anything about AI are just saying that they do AI. I was on a panel recently in NY at one of the events and a speaker said, “I say I do AI so that I can increase my money.” AI has become a trend; even if you don’t know it, you say you do AI, and you get more funding, or you get more clients.
AI is going to have real impact in a lot of industries - auto industry, customer service, finance, banking (having machines predict who should get what amount of loan), construction (hydropower and building) and insurance. We are working with a client who is trying to do predictive insurance. So in the US, a regular driving car, when you turn it on, it starts to emit quite a lot of data points every second which tracks your driving skill - are you braking too hard, are you turning right too fast without slowing down. The machine takes all of that and predicts if the person should get insurance at $100 a month or $50 a month.
Do you think Nepal is ready for technology entrepreneurship?
Nepal is definitely ready for technology and entrepreneurship. Is Nepal ready for AI, is a different question. I think ‘Nepal getting ready for AI’ is almost there but one of the biggest hurdles for AI deployment at a country level or being deployed across many industries in a country is the infrastructure related to data. I am not talking about infrastructure with roads, and cars and electricity, but for AI to work, it needs a lot of data. You will have a lot of data for a particular industry, if you have been collecting and storing it. But in Nepal, that’s not the case. A lot of things get done on paper but they are never digitized. There are a lot of processes where nothing really gets tracked which means there is a lot of data infrastructure that the government needs to create or the companies need to create within themselves so that when somebody wants to say “I want to implement this algorithm to automate this task, the data is all together.” But that’s not the case so that’s why it is going to take a little bit more time for Nepal than for some other few countries.
Text: Ujeena Rana Photos : Indepth Photography